Hawes Hall, Classroom 201, Harvard Business School
Topic: Towards Causal Artificial Intelligence
Causal inference is usually dichotomized into two categories, experimental (Fisher, Cox, Cochran) and observational (Neyman, Rubin, Robins, Dawid, Pearl) which, by and large, are studied separately. Understanding reality is more demanding. Experimental and observational studies are but two extremes of a rich spectrum of research designs that generate the bulk of the data available in practical, large-scale situations. In typical medical explorations, for example, data from multiple observations and experiments are collected, coming from distinct experimental setups, different sampling conditions, and heterogeneous populations.
In this talk, I will introduce the data-fusion problem, which is concerned with piecing together multiple datasets collected under heterogeneous conditions (to be defined) so as to obtain valid answers to queries of interest. The availability of multiple heterogeneous datasets presents new opportunities to causal analysts since the knowledge that can be acquired from combined data would not be possible from any individual source alone. However, the biases that emerge in heterogeneous environments require new analytical tools. Some of these biases, including confounding, sampling selection, and cross-population biases, have been addressed in isolation, largely in restricted parametric models. I will present my work on a general, non-parametric framework for handling these biases and, ultimately, a theoretical solution to the problem of fusion in causal inference tasks.
Suggested readings:
- Causal inference and the Data-Fusion Problem
- Causal Inference and Data-Fusion in Econometrics
- On Pearl’s Hierarchy and the Foundations of Causal Inference
The reading group will meet at 2PM before the seminar.
Discussant: Larry Han
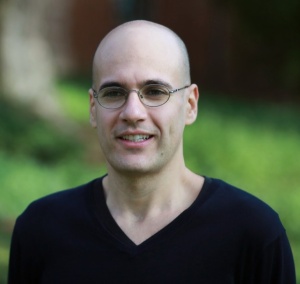
Elias Bareinboim
Associate Professor of Computer Science
Columbia University
The Fu Foundation School of Engineering and Applied Science